Overcoming Materials Synthesis Challenges with Data and AI at Scale
October 11, 2024
Blog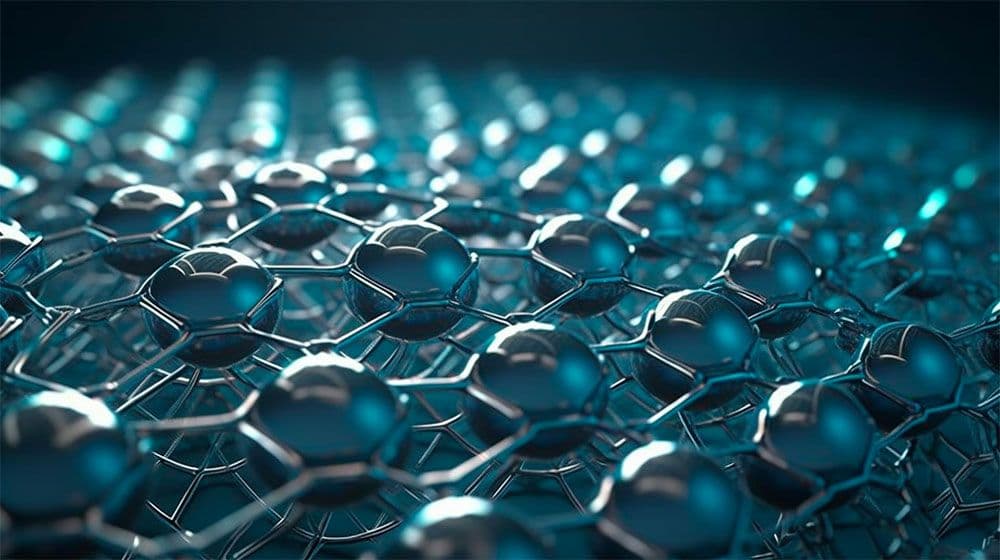
Next-generation technologies depend on the improvement of existing materials platforms and the development and rapid commercialization of new ones. In fields spanning semiconductors, batteries, communications, quantum computing, and many others, the search for greater performance is shifting from one-material-fits-all monolithic development to application-specific optimized materials platforms. This shift is driven by more diverse performance requirements of new applications and physical limits being reached in existing materials platforms. However, making new materials at lab, prototype, and production scales is deeply challenging due to complex, nonlinear underlying dynamics which are strongly coupled to individual manufacturing hardware. As a result, the commercialization of new materials is historically slow.
Like other areas of manufacturing, there is significant potential to leverage modern sensor and process data streams generating large-scale data with application-specific artificial intelligence (AI) and machine learning (ML) to improve the efficiency of recipe design, process scale-up, and quality control. In applying AI to advanced materials synthesis, there are several unique challenges to overcome which can unlock a vast opportunity space for innovation and rapid commercialization.The Potential of Data-Driven Synthesis Control
In advanced materials, outsized performance and reliability gains are made by controlling materials processing with high precision. High resolution observations generating large data volumes are necessary to achieving the precision required to manage these complex processes. Traditional approaches to interpret this data and adjust materials processing rely on practitioner intuition and trial and error, placing a hard ceiling on the throughput for process optimization. Data-driven techniques can automate some of these steps, to leverage the explosion of data from modern metrology (the science of measurement) and characterization tools. If end-to-end datasets spanning recipe metrics (e.g. temperatures, pressures, precursors, fluxes) and high-resolution materials outputs can be consistently assembled, tailored AI/ML techniques can be applied to model the nonlinear synthesis relationships and massively accelerate the feedback loop.
To fully capitalize on this potential, organizations need the right infrastructure and capabilities. We believe that overcoming the challenges of nonlinear synthesis control requires a holistic approach, which includes:- Building Intelligent Automations to Generate Foundational Datasets
- The first step is to create automated systems capable of extracting effective "information" from raw observation data without human intervention.
- Automation in metrology and characterization will enable the generation of foundational datasets at scale, reducing manual intervention and enabling high-throughput experiments.
- These datasets provide the raw material needed for AI/ML models to learn and infer patterns in the synthesis process.
- Such automation will enable real-time monitoring of material processing workflows, offering high-resolution insights into how process variables impact material properties.
- Creating a Integrated Data Ecosystem to Enable Use of Large-Scale AI Models
- To maximize the value of their data, organizations need a fully integrated data ecosystem that captures, stores, processes, and analyzes data throughout the entire synthesis and testing cycle.
- This ecosystem must link data from various stages of the processing life cycle, including equipment and environmental data, recipe, metrology, characterization, and performance data.
- Centralizing this data allows for large-scale inference, where AI models can assess the relationships between process parameters and resulting materials properties, as well as optimize the overall experimental design and search pattern.
- Such a system provides a feedback loop that accelerates knowledge discovery and enables more efficient experimentation and optimization.
- Accelerating Feedback Loops for Real-Time Control and Optimization
- One of the most promising aspects of automated materials data inference is to enable real-time feedback during the synthesis process, allowing engineers to adjust parameters on the fly.
- Real-time feedback, driven by AI, would enable continuous process optimization, where synthesis conditions are adjusted in real-time based on predicted outcomes. This could drastically reduce the time needed to achieve optimal material properties and speeds up the commercialization process.
- Intelligent trial design, powered by adaptive sampling and prediction, can reduce the number of iterations required by targeting the most promising process configurations.